Is culture the key to AI's next big breakthrough?
AI has yet to reckon with the social forces that shape intelligence.
The field of artificial intelligence has often used what we know about human intelligence as a template for designing its systems. But for the most part, AI has always drawn on a view of the mind in social isolation, disconnected from the community of other minds. Yet one of the big insights of modern psychology—for example in work on embodied cognition—is that thinking doesn’t just take place between an individual’s ears. Human cognition is shaped by social and culture forces. What might it look like for AI models to take these forces into account?
AI as the search for fundamental principles of intelligence
In general, there are two ways to think about the development of modern artificial intelligence. The first is that AI is an exercise in engineering. A given algorithm either works or it doesn’t, and whether it works isn’t necessarily indicative of any deeper underlying principles in any scientific sense. The guiding metaphor for this view is aviation. We didn’t invent airplanes by studying birds. We started from scratch, without looking to evolution for inspiration, and simply tried different things until some of them started to work. But this isn’t the main sentiment among researchers in AI. Throughout the history of AI, its practitioners have always suspected that what they’re engaged in something deeper, that their work is uncovering something more profound. In this view, AI is not just engineering problem. It is the search for the fundamental principles of intelligence.
As a consequence, the influence between what we know about AI and what we know about human cognition is bidirectional. By formalizing their theories with models from AI, cognitive scientists can go beyond the box-and-arrow diagrams of their field and actually show whether what they know about cognition can be used to build a system with human-like thinking capabilities.
In the other direction, insights about human intelligence have tended to trickle down into AI—having sat around for a while with unappreciated utility and used at length by people in only in their vaguest, most general forms. This began long before the invention of modern computers. For example, George Boole tried to formalize human reason in his 1854 treatise on mathematical logic, The Laws of Thought. This work was based on the oldest insight in the psychological book—that man is a rational animal—and, as there was not yet a formal field of psychology in Boole’s time, he built on the Enlightenment’s renewed enthusiasm in reasoning and rationality.
The cycle of insight to application has turned with increasing speed over the years, given that the transfer of knowledge moves at an exponential clip. But one way to interpret the ideas in AI in the middle of the 20th century are as a response to ideas from psychology and neuroscience at the beginning of that century. For example, in his 1950 paper, Computing Machinery and Intelligence, Alan Turing posed the question, “Can machines think?” The dominant insights in psychology in the early 1900s, prior to the establishment of Behaviorism in 1913 by John Watson, came from William James: the stream of consciousness. Turing was, in a sense, asking whether it made sense to apply the same concept to computers. He called his proposed method for answering this question the imitation game; we know it as the Turing Test.
Perhaps slightly less well-known, but no less central, was John von Neumann. Besides the Turing Test, Turing’s other enduring invention was the Turing Machine. This was not actually a machine, but a theoretical construct showing what it would look like to create a “universal computer.” Essentially: a program that could designed to run other programs. Von Neumann used the theoretical work by Turing as a basis for the architectural design of the modern computer. Turing showed a computer was possible in theory; von Neumann actually made one. In his 1958 monograph, The Computer and The Brain, von Neumann speculates that, some important differences aside, the machine he built with digital circuits appeared to work in a similar way to the neural circuits of the brain. What were his insights about the workings of neurons based on? Little more than what could be inferred from the pioneering work of Santiago Ramón y Cajal.
Likewise, the first chess playing programs were designed by cognitive scientists intent on modeling the strategies of expert chess players (Herb Simon had to write the programs out by hand, because contemporary computers weren’t sophisticated enough to run them). Artificial neural networks—the precursor for today’s deep learning—were designed to address some of the differences pointed out by von Neumann. And reinforcement learning, through which an AI program interacts with an environment and learn from feedback, was based on the stimulus-response theories of Behaviorist psychology.
The overall pattern is that a lot of the central paradigms in AI are based on psychological insights, but only very generally. They are based on a true story—but with the caveat that the adapter is importing the story from another language, one she doesn’t really speak, and at any rate is way more focused on the kernel of wisdom that makes the story compelling rather than maintaining fidelity to the details of the original work.
In much the same way, the concept of Artificial General Intelligence—AI’s holy grail—is only loosely based on an understanding of what it means to be an agent with the full breadth of human cognitive abilities. Specifically, flexibility. What AGI is after is flexible cognition. Because a single human mind can learn to use language, perform complex reasoning, write computer programs, write poetry, tell you that a pound of feathers weighs the same as a pound of steel, and solve problems from architecting skyscrapers to navigating across open sea—then it must be possible to design an AI that can do so as well.
AI’s biggest and best paradigms come from looking at human cognition, but from very far away and only with any eye for general patterns that might be well-suited to implementation in computer programs.
So it makes sense to ask the question: What is the most general insight from psychology and cognitive science that is under-appreciated by people in AI?
Many cognitive scientists are happy to provide answers to this question. For example, Gary Marcus has been on a decades-long quest to disbelieve that progress in AI is really happening. But what I take to be the orthodoxy is a 2017 paper by researchers at Harvard, NYU, and MIT (for the record, I’m biased on what counts as the central beliefs of the field; I was working in one of their labs at the time). But paper focuses on specific things that need to be built into AI systems. Their goal is to say, if you really understood how the mind works and wanted to construct your AI accordingly, here is what you’d do.
I want to try to address a different question. What if you didn’t really understand the cutting edge of what was happening in cognitive science? What if you were, say, 20 years behind what was happening in the field, with only a rudimentary ability to appreciate what these insights mean? What if you stood really far away from the sum total knowledge of the field and just sort of squinted at it? After all, this is what the lineage of Boole and Turing and von Neumann and Simon and all the other paradigm-makers of AI seemed to have done.
Suppose you did that. What would you see?
The mind: a view from far away
What you would see, I think, is that human cognition is a more social phenomenon than we previously gave it credit for. What AI people see when they look at intelligence is a lone individual—probably a computer programmer or a mathematician; definitely a genius—sitting alone in a room, with the full resources of human knowledge at their finger tips, synthesizing and applying this knowledge in ways no one had ever thought to do before. And, according to this lone-genius view of intelligence, the fact that human beings are social creatures is entirely separate from the fact that they are smart ones.
To be fair to AI people, this belief goes beyond the walls of computer science departments to the heart of Western philosophy. This is what Boole, inspired by Enlightenment thought, has in common with modern AI: the belief that we are the fundamental processes that make up our mind. It is for this reason that we named our own species Homo sapiens (wise man), rather than Homo cooperans (cooperative man), and endorse statements as I Think, Therefore I Am. In this view, the fundamental unit of intelligence has always been the individual. Not the neuron. Not the species. Not the tribe. But the lone-genuis sitting at her desk, typing away in the dark. It is easy to forget that this is not a fact, but a framework.
As the anthropologist Clifford Geertz once wrote:
The Western conception of the person as a bounded, unique, more or less integrated motivational and cognitive universe, a dynamic center of awareness, emotion, judgment, and action organized into a distinctive whole and set contrastively both against other such wholes and against its social and natural background, is, however incorrigible it may seem to us, a rather peculiar idea within the context of the world’s cultures.
The point is that people in AI think they will develop AGI by recreating a single human-like mind: one deep neural network to rule them all. But I don’t think that’s right. The problem with trying to follow the formula laid out by leading cognitive scientists I cited above, eminently reasonable and defensible though it is, is that AI researchers will hit the same ceiling they did when they tried to code systems for natural language processing by hand. There are just too many things to include in the system to get it to work. How do you know what to include, when to stop?
Here’s my from-a-distance version of what I think cognitive science tells us to do instead: Humans aren’t born terribly smart. They become smart by interacting with other humans. To be sure, humans are definitely born with some innate cognitive structure, that much is obvious and well-documented in developmental psychology. But the inescapable fact is that newborn human babies are helpless, physically and cognitively, in just about every possible respect. They have to do a lot of learning—and even though they get to from here to there eventually, they start from a point of pretty blatant incompetence.
The field of AI has already learned a version of this once, when they realized the importance of data. In the early years of AI, researchers concerned themselves largely with internal structure. This was the impulse, for example, of so-called expert system approaches, which tried to bake in as much concrete human knowledge to their architecture they could pack into it. But the approach didn’t scale. The biggest breakthrough in modern AI—going from AI being almost entirely useless to actually quite useful—was the development of deep learning. Yet this paradigm largely resembles the technical specifications for the neural nets dreamt up by cognitive scientists like David Rumelhart and Jay McLelland in the 1980s. It was only when it became possible for these systems to process a lot more data that they achieved the impressive results of modern deep learning. It wasn’t the internal structure of the model that mattered. It was what was going on outside of it.
The big insight of modern cognitive science is that the same is true of the human mind. Internal structure is definitely important. But the mind is more than just its fundamental processes. How that mind works depends largely on the world that mind inhabits. In particular, its social and cultural milieu.
To use the computer metaphor of the mind favored by many cognitive scientists and people in AI: trying to understand a mind in the absence of a larger social context is like trying to understand a laptop without realizing it is supposed to be connected to the internet. The laptop can do an awful lot without having to be connected to the cloud. You can create a document in a word processor; you can record a song in an audio editor; you can search through the files on the hard drive; you can even write a new program for the machine to run. But you will also miss a lot of the functionality that defines how people typically use the device. You will open the web browser and conclude this program is basically useless. You will think the video calling app is designed mainly to check your own appearance in the camera. You will suppose that the number of songs that can be played by this machine is quite limited. And you will have a hard time imagining what to do next with that document you’ve written or the song you’ve recorded besides turning the screen around and showing it to the colleague sitting across from you. In short, you will be able to understand a great deal about how that system works, and you might even be impressed by its capabilities. But you will fall dramatically short of appreciating the full breadth of what it can do.
AI models don’t need to be terribly smart. They need to become smart by interacting with other AI models. In a word, they need a mechanism for learning via culture.
The social foundations of human intelligence
Specifically, there is a prominent theory from a cognitive scientist named Michael Tomasello which makes a prediction about what exactly it is about culture that is crucial for the development of human intelligence.
Tomasello’s academic advisor was a man named Jerome Bruner, who was an influential early cognitive scientist but skeptical of computer metaphor of the mind. Bruner’s research focused primarily on developmental psychology, and so Tomasello started off studying infant behavior. But Tomasello’s personal interest was in apes. In particular, he wanted to figure out what it was about human infants that allowed them to grow into fully-fledged adults, capable of learning human languages and participating in society, in the way that chimps, for all their sophisticated cognitive and social skills, could never do.
Tomasello presents his most influential theory based on two decades of work, published in 2000, in his book The Cultural Origins of Human Cognition. Tomasello opens the book with a puzzle, one based on the archeological record of human evolution.
According the best available estimates, humans split from the other great apes about six million years ago. Two million years after that, the genus Homo emerged. Initially, there were multiple species of Homo. But then, suddenly, around 200,000 years ago, one population of Homo began outcompeting all the others. Their descendants became Homo sapiens: cognitively modern humans.
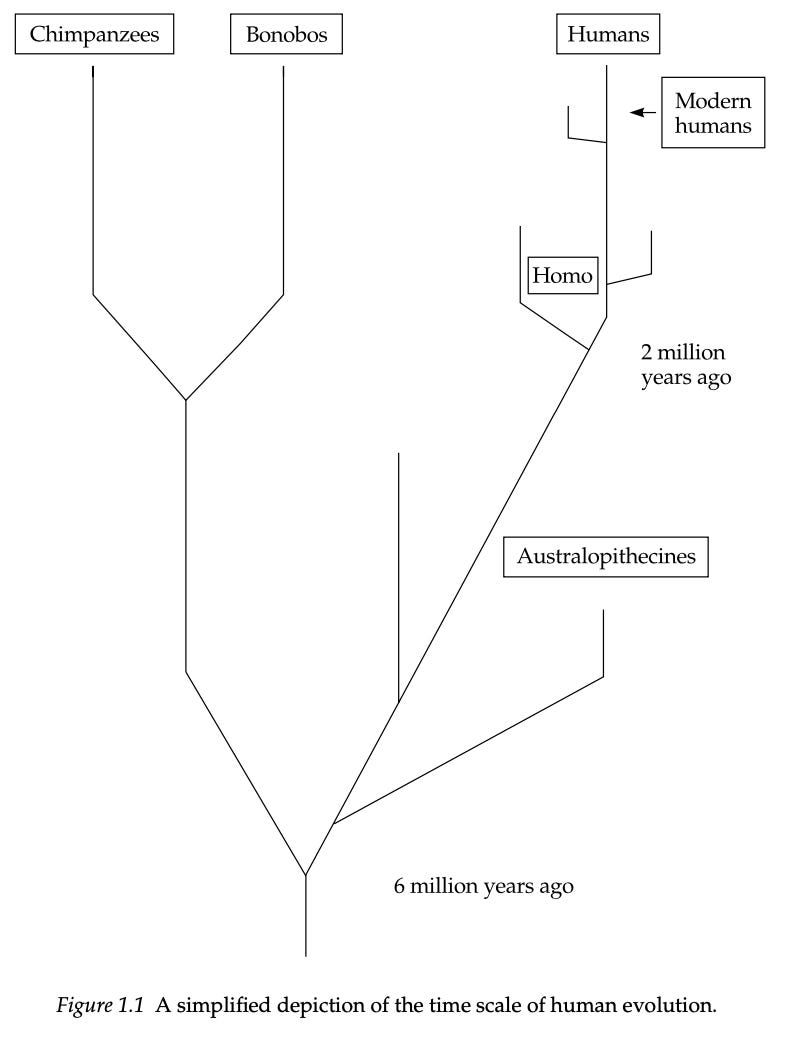
What happened during this course of evolution that allowed the human side of the branch to build cities and farms and Facebook, while chimpanzees and bonobos remained in the trees? Tomasello points out that this adaptation happened in an incredibly short amount of time by evolutionary standards. After all, humans and chimps share the same amount of genetic material as rats and mice, lions and tigers, and horses and zebra. But the difference in outcomes, civilization building-wise, between humans and chimps is far greater than those between rats and mice.
“Our problem,” as Tomasello writes, “is thus one of time.”
The fact is, there simply has not been enough time for normal processes of biological evolution involving genetic variation and natural selection to have created, one by one, each of the cognitive skills necessary for modern humans to invent and maintain complex tool-use industries and technologies, complex forms of symbolic communication and representation, and complex social organizations and institutions.
In other words, Tomasello’s argument is that, overall, the fundamental processes of human cognition must be very similar to that of our great ape ancestors. There wasn’t enough time for evolution to patch together an entirely new set of cognitive processes. Instead there must have been a small number of cognitive innovations—say, one or two—which allows humans to leverage the capacities of their otherwise ape-like minds in new ways. This cognitive innovation, then, is a kind of cognitive missing link—leading from our great ape ancestors to cognitively modern humans—which allowed early Homo sapiens to accumulate, generation over generation, the cultural knowledge that modern civilization is built on.
Tomasello describes the mechanism behind this cognitive missing link as the “cultural ratchet.” Decades of research had already shown that non-human primates were capable of innovative tool use, crucially in work by Jane Goodall and her colleagues in the 1980s. The problem was slippage. A chimp might come up with a new way of using a stick to get food. But other chimps wouldn’t notice the effectiveness of the new behavior and appropriate it for themselves, as would be natural for humans. In order for a species to accumulate cultural knowledge over time, they need both the creativity component and the stabilizing ratchet component.
In other words, human intelligence isn’t just about the processes inherent in a single mind. It reflects our species’ unique ability to, as the quotation goes, stand on the shoulders of giants. What we think of as the modern human mind is a single cross-sectional moment in the upward trajectory of human intelligence. It is the accumulation of thousands of generations of our ancestors, each turning the cultural ratchet just a bit, and passing on the best of their insights and innovations to the next generation. This, for example, is one explanation for the Flynn Effect, the empirical observation that our species’ average score on intelligence tests tends to go up over time. Without the ability to accumulate cultural knowledge, there would be no cultural ratchet. And without the turning of the cultural ratchet, we would not have human cognition as we understand it today.
So what might this mean for pursuit of AGI, an artificially intelligent agent with human-like cognitive capabilities? In short, it means that even if we did replicate the fundamental processes of human cognition, it wouldn’t be enough. It would be like creating a laptop without the ability to connect to the internet. This machine would have a lot of useful functionality. But it would fall short what we know that technology is potentially capable of doing.
If Tomasello’s theory holds water, then human-like intelligence has two ingredients. The first would be the fundamental processes that underlie human cognition—including the cognitive ability to turn the cultural ratchet. The second ingredient is an opportunity to actually turn it. In other words, to engage in the kind of generation-over-generation learning that led to contemporary human intelligence.
As Tomasello wrote in a 2014 update to his theory, called A Natural History of Human Thinking:
Human adults are cleverer than other apes at everything not because they possess an adaptation for greater general intelligence but, rather, because they grew up as children using their special skills of social cognition to cooperate, communicate, and socially learn all kinds of new things from others in their culture, including the use of all of their various artifacts and symbols.
We typically think that what allows humans to be social is their general intelligence. But what if we have it backwards? Typically, we think that our species’ increased intelligence caused us to become social creatures. If this is the case, then the fact that we are social creatures really is divorced from our being smart ones. There is no reason that sociality is a necessary ingredient in intelligence. But according to Tomasello’s theory, we have the causality wrong: we are intelligence creatures specifically because we are social ones. What we perceive as human intelligence—yes, even the lone genius alone in her room—is the result not just of the fundamental processes of the mind, but thousands of generational turns of the cultural ratchet.
As the developmental psychologist Jean Piaget once wrote, “Only cooperation constitutes a process that can produce reason.” AI is a search for the beating heart of reason. But, as it stands, it doesn’t think it needs cooperation to get there.
Artificial Collaborative Intelligence
If this is true, that human-like cognition is only possible via social mechanisms, then what the field of AI should be aiming for is less like AGI and more like ACI: Artificial Collaborative Intelligence.
For example, the current approaches in AI spawn ChatGPT, a chatbot which can produce language at a human level. But imagine: What if ChatGPT had the ability to talk with other ChatGPTs? What if it could learn from these conversations, the same way that one human can learn from talking to another? Unlike human conversation, this dialogue wouldn’t be limited by the speed of human reading or speaking or even thought. These two ChatGPTs would be able to analyze the entirety of human knowledge, going back and forth on what to make of it, with essentially no bounds on the depth of that scrutiny.
Now imagine that kind of conversational ability on the scale of thousands of ChatGPTs. They would all be engaged in discussion simultaneously and sharing their insights centrally, like a condensed version of all the world’s universities, think-tanks, and institutes combining their collective intellectual power.
This version of Artificial Collaborative Intelligence would replicate the way human learning works across historical time. New data comes to light, and new ideas and insights are proposed; these insights are debated and refined. The best of them are taken up by the next generation, and the ones that don’t work are replaced by new ideas. This evolutionary process would yield the kind of intelligence we think of truly human in a way that emulating the mind’s fundamental processes never could. But with ACI, it would occur on an unprecedented scale: each agent with its own ability to consult, analyze, and communicate its perspective on the sum total of human knowledge. At lightning speed, this perspective gets checked by the perspective of a potentially unlimited number of other agents engaged in the same process. It is difficult not to see this as the AI breakthrough of the future.
It is also worth noting that in the cases where a preliminary version of this approach has been tried, it is has been a huge success.
The most obvious example is AlphaGo playing against itself. In the original version of the program, developed by Google Deep Mind, the AI program was trained on a data set including thousands of previously played matches. This was enough to go toe-to-toe with the leading human Go player, Lee Sedol. However, the real breakthrough came with AlphaGo playing games not against human players—but against itself. It was then able to play a functionally infinite number of games, turning the cultural ratchet on its own ideas. As another Go champion said of these matches: they were like “nothing I’ve ever seen before - they’re how I imagine games from far in the future.”
A more subtle example is AlphaFold. This is Deep Mind’s program for predicting the structure of unknown protein molecules. The key innovation was creating a program that could not just learn from examples of known proteins, but generate predictions of what unknown proteins might be discovered. These hypothetical proteins were used as examples for another program to learn from, the way a teacher might come up with example problems to give to their students. It was only with this approach that the program was able to predict novel protein structures down to an atomic level of accuracy.
Personally, I think the reason that AI hasn’t spent much time considering an ACI framework is that it runs against the field’s most deeply held philosophical assumptions. If the mind is its own bounded cognitive universe, there is no principled reason why multiple minds would be needed to create intelligence. With Tomasello’s theory, it becomes clear why multiple minds and a mechanism for cultural learning is necessary. If people want AI to have more intelligence, what they need is not to build AI systems with a greater capacity for intelligence. It is to build them with a greater capacity to engage with one another. AI needs a mechanism for culture.
I mean, letting AI build its own culture. What could go wrong?
Interesting insight on human development and AI.
"Learning, no matter how much, will not teach a person to understand." (Heraclitus, 450 BC)
AI learns the same way the animals learn, the same way humans learn -- because we are, in part, AIs. What AI cannot do is to understand. To be sure, humans too have been struggling:
"Even though the Logos [the Understanding] always holds true, people fail to comprehend it even after they have been told about it." (Heraclitus)
"In [the Logos] was life, and the life was the light of men. And the light in the darkness shined; and the darkness comprehended it not." (John 1:5)
And we pay dearly for our confusion: “No man chooses evil because it is evil; he only mistakes it for happiness, the good he seeks.” (Mary Wollstonecraft)
The reason, we struggle to develop the capacity for understanding is that we don't teach it in schools. We dump knowledge on children hoping that they will somehow figure out what to do with it. And a few would discover that capacity in them, but most won't, and they will live their lives pretty much as AIs. Still, at least we all have the potential for understanding.
AI, in its current incarnation, may simply lack the hardware. But even that aside, how could we teach AI to understand (or give it the necessary hardware) when we don't know how to awake this capacity, with any consistency, in our own children? Even though human ancestors had spent five million years evolving it.